Exploring Machine Learning: Comprehensive Insights and Applications
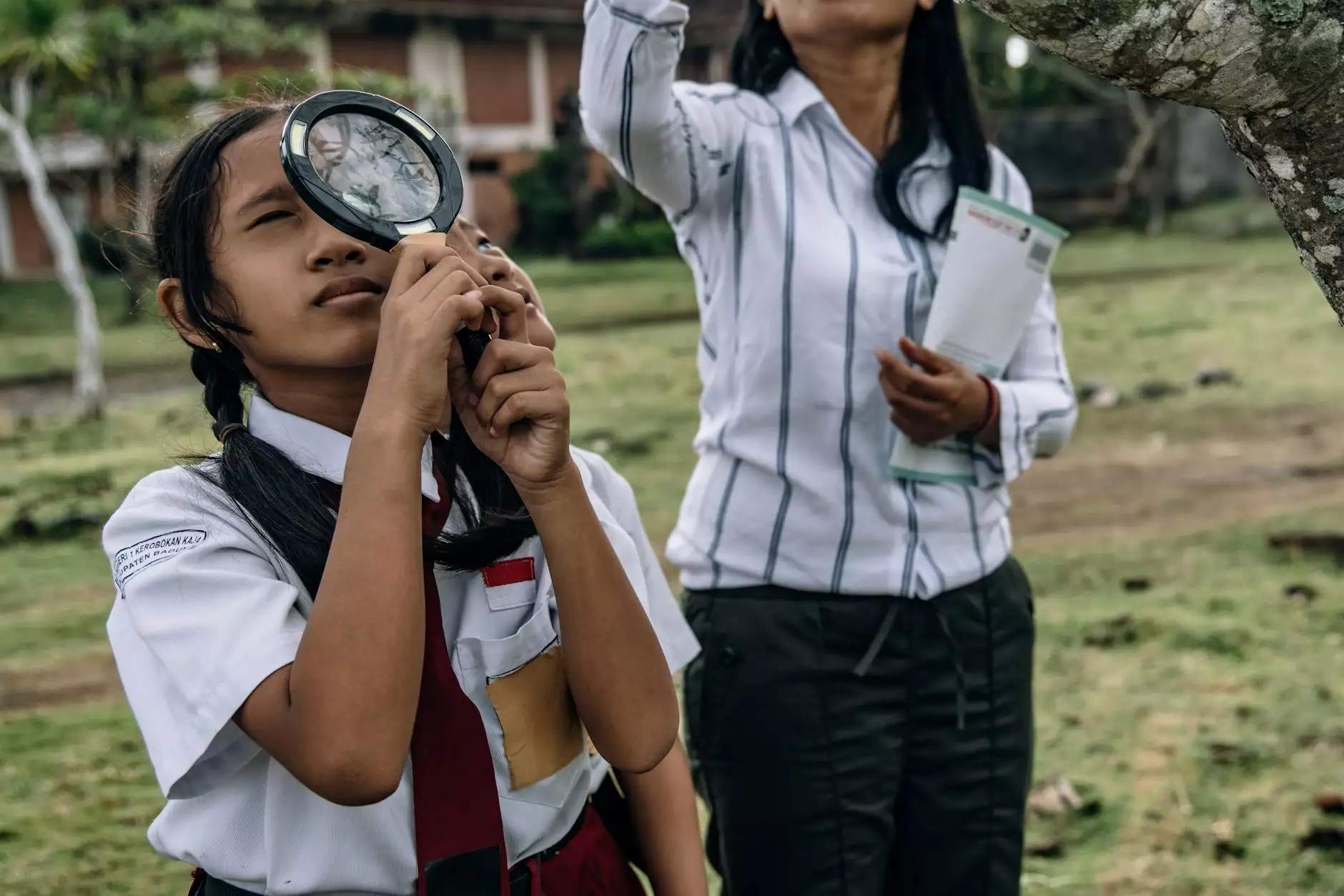
Machine learning has emerged as one of the most transformative technologies in modern times. From analyzing vast amounts of data to automating complex processes, machine learning continues to revolutionize industries and reshape how businesses function. In this extensive article, we will delve into various aspects of machine learning, explore its applications, and discuss the significance of comprehensive articles about machine learning for businesses and professionals alike.
Understanding the Essentials of Machine Learning
At its core, machine learning is a subset of artificial intelligence (AI) that focuses on the development of algorithms that allow computers to learn from and make predictions based on data. Unlike traditional programming, where developers write explicit instructions, machine learning systems learn directly from data and improve over time, enhancing their accuracy and performance.
Types of Machine Learning
Machine learning can be broadly classified into three main categories:
- Supervised Learning: This type involves training a model on a labeled dataset, where the output is known. The system learns to map input data to the desired output and can make predictions on new, unseen data.
- Unsupervised Learning: In this approach, the model is provided with data that does not have labeled responses. The system tries to identify patterns and structures within the data. Clustering and association are common techniques used in unsupervised learning.
- Reinforcement Learning: This method is inspired by behavioral psychology, where an agent learns to make decisions by taking actions in an environment to maximize some notion of cumulative reward. It is widely used in robotics, gaming, and navigation.
Key Components of Machine Learning
To effectively utilize machine learning, one must understand its critical components:
- Data: Quality and quantity of data are paramount. Machine learning models require large datasets to train effectively, making access to high-quality data a key factor in their success.
- Algorithms: The algorithms determine how the model learns from the data. Different algorithms can lead to varied outcomes, making the choice of algorithm crucial.
- Model Training: This process involves feeding the data into the algorithm and allowing it to learn and adapt. The training phase is essential for ensuring that the model can perform accurately when faced with new data.
- Evaluation: After training, the model must be evaluated using metrics such as accuracy, precision, recall, and F1 score to ensure it performs well in real-world scenarios.
The Importance of Articles About Machine Learning for Businesses
Understanding and implementing machine learning can provide businesses with a significant competitive advantage. Engaging with high-quality articles about machine learning can help professionals stay ahead of the curve. Here’s why such resources are indispensable:
- Knowledge Enhancement: Articles provide insights into the latest research trends, technologies, and methodologies, enriching a professional's knowledge base.
- Practical Applications: Well-written articles demonstrate real-world applications of machine learning, helping businesses translate theory into practice.
- Best Practices: Learning from the successes and challenges of others helps businesses develop best practices for implementing machine learning initiatives.
- Networking Opportunities: Articles often cover conferences, webinars, and communities which can open doors for networking and collaboration.
Transformative Applications of Machine Learning Across Industries
Machine learning is not limited to any single industry; its applications span various sectors, each benefiting uniquely from its capabilities. Here are several prominent areas where machine learning is making a significant impact:
1. Healthcare
In the healthcare sector, machine learning algorithms are used to analyze patient data, leading to improved diagnosis and treatment options. Applications include:
- Predictive Analytics: Predicting patient outcomes based on historical data can lead to proactive care and better health management.
- Medical Imaging: Machine learning techniques enhance image analysis, aiding in the identification of diseases such as cancer.
- Personalized Medicine: Tailoring treatment plans to individual patients based on their genetic makeup and responses to medication is becoming increasingly feasible.
2. Finance
Machine learning in finance helps improve risk management, fraud detection, and customer service. Key applications include:
- Algorithmic Trading: Trading algorithms based on market patterns can automate trading processes and maximize profits.
- Credit Scoring: Machine learning models enhance the accuracy of credit risk assessments by analyzing a wide range of customer data.
- Fraud Detection: Identifying unusual patterns in transaction data allows financial institutions to detect and prevent fraudulent activities.
3. Retail
In the retail industry, machine learning can greatly enhance customer experience and operational efficiency. Notable applications include:
- Customer Personalization: Analyzing purchase history and preferences enables retailers to offer personalized recommendations.
- Inventory Management: Predictive analytics can optimize stock levels and supply chain logistics by forecasting demand trends.
- Sentiment Analysis: Understanding customer feedback through sentiment analysis can drive improvements in products and services.
4. Transportation
Machine learning is at the forefront of innovating transportation systems. Here are some applications:
- Autonomous Vehicles: ML algorithms are essential for enabling self-driving cars to navigate and make real-time decisions.
- Route Optimization: Analyzing traffic patterns helps in planning efficient transit routes to reduce congestion and travel time.
- Predictive Maintenance: Machine learning predicts vehicle maintenance needs based on usage data, enhancing safety and reducing costs.
5. Marketing
In marketing, machine learning algorithms predict consumer behavior and enhance targeting strategies. Important applications include:
- Customer Segmentation: Automating the segmentation process allows marketers to target specific groups more effectively.
- Churn Prediction: Identifying at-risk customers aids in developing retention strategies to improve loyalty.
- Ad Optimization: Analyzing the performance of various ad campaigns can inform adjustments and allocate budgets more effectively.
The Future of Machine Learning
The journey of machine learning is just beginning, and its future holds immense promise. As businesses continue to adopt and integrate machine learning solutions, the following trends are expected:
- Ethical AI: As companies navigate ethical dilemmas associated with AI, discussions around responsible AI practices are becoming increasingly important. Ensuring fairness, transparency, and accountability in machine learning algorithms will be paramount.
- Automated Machine Learning (AutoML): Tools that automate the machine learning workflow will make it simpler for non-experts to leverage ML technologies and drive innovation.
- AI Democratization: With increasing access to machine learning tools and resources, a broader base of individuals and organizations will be able to harness the power of machine learning. This democratization will enable innovation across industries.
- Continued Research: Ongoing research will lead to advancements in algorithm effectiveness, interpretability, and efficiency, expanding the scope of what is feasible with machine learning.
Conclusion
Engaging with quality articles about machine learning is essential for professionals and businesses looking to thrive in the data-driven world. By understanding the nuances of machine learning, its applications, and the broader implications for the industry, one can unlock new opportunities for growth and innovation. As machine learning continues to evolve, so too will its role in shaping the future of technology and business. Embracing this transformative journey is not only wise but necessary for long-term success.